Online Instructor-Led Certification BootCamp
DP-203: Data Engineering on Microsoft Azure
5 Days Instructor-led training
In this course, the students will design various data platform technologies into solutions that are in line with business and technical requirements. This can include on-premises, cloud, and hybrid data scenarios which incorporate relational, NoSQL, or Data Warehouse data. They will also learn how to design process architectures using a range of technologies for both streaming and batch data.
The students will also explore how to design data security, including data access, data policies, and standards. They will also design Azure data solutions, which includes the optimization, availability, and disaster recovery of big data, batch processing, and streaming data solutions.
In this course you will learn to:
- Learn how to design and build secure, scalable, and performant solutions in Azure by examining the core principles found in every good architecture
- Learn how using key principles throughout the architecture, regardless of technology choice, can help you design, build, and continuously improve the architecture for an organization’s benefit
- Learn the reference design and architecture patterns for dealing with the batch processing of data
- Will also be exposed to an AI architecture and how the data platform can integrate with an AI solution
- Learn the reference design and architecture patterns for dealing with streaming data
- Explore a data science architecture that streams data into Azure Databricks to perform trend analysis
- Learn how an Internet of Things (IoT) architecture will require data platform technologies to store data
- Learn how to incorporate security into an architecture design and learn the key decision points in Azure provided to help create a secure environment through all the layers of architecture
- Learn scaling services to handle the load. They will learn how identifying network bottlenecks and optimizing storage performance
- Learn how to handle infrastructure and service failure, recover from the loss of data, and recover from a disaster
- Learn how to design an Azure architecture that is operationally-efficient and minimizes costs by reducing spend
- Understand how to design architectures that eliminate waste
The audience for this course is Data Professionals, Data Architects, and Business Intelligence Professionals who want to learn about the data platform technologies that exist on Microsoft Azure. The secondary audience for this course is individuals who develop applications that deliver content from the data platform technologies that exist on Microsoft Azure.
Job role: Data Engineer
Module 1: Explore compute and storage options for data engineering workloads
This module provides an overview of the Azure compute and storage technology options that are available to data engineers building analytical workloads
-
- Introduction to Azure Synapse Analytics
- Describe Azure Databricks
- Introduction to Azure Data Lake storage
- Describe Delta Lake architecture
- Work with data streams by using Azure Stream Analytics
Lab: Explore compute and storage options for data engineering workloads
-
- Combine streaming and batch processing with a single pipeline
- Organize the data lake into levels of file transformation
- Index data lake storage for query and workload acceleration
Module 2: Design and implement the serving layer
This module teaches how to design and implement data stores in a modern data warehouse to optimize analytical workloads.
-
- Design a multidimensional schema to optimize analytical workloads
- Code-free transformation at scale with Azure Data Factory
- Populate slowly changing dimensions in Azure Synapse Analytics pipelines
Lab: Designing and Implementing the Serving Layer
-
- Design a star schema for analytical workloads
- Populate slowly changing dimensions with Azure Data Factory and mapping data flows
Module 3: Data engineering considerations for source files
This module explores data engineering considerations that are common when loading data into a modern data warehouse analytical from files stored in an Azure Data lake and understanding the security consideration associated with storing files stored in the data lake.
-
- Design a Modern Data Warehouse using Azure Synapse Analytics
- Secure a data warehouse in Azure Synapse Analytics
Lab: Data engineering considerations
-
- Managing files in an Azure data lake
- Securing files stored in an Azure data lake
Module 4: Run interactive queries using Azure Synapse Analytics serverless SQL pools
In this module, students will learn how to work with files stored in the data lake and external file sources, through T-SQL statements executed by a serverless SQL pool in Azure Synapse Analytics. Students will query Parquet files stored in a data lake, as well as CSV files stored in an external data store. Next, they will create Azure Active Directory security groups and enforce access to files in the data lake through Role-Based Access Control (RBAC) and Access Control Lists (ACLs).
-
- Explore Azure Synapse serverless SQL pools capabilities
- Query data in the lake using Azure Synapse serverless SQL pools
- Create metadata objects in Azure Synapse serverless SQL pools
- Secure data and manage users in Azure Synapse serverless SQL pools
Lab: Run interactive queries using serverless SQL pools
-
- Query Parquet data with serverless SQL pools
- Create external tables for Parquet and CSV files
- Create views with serverless SQL pools
- Secure access to data in a data lake when using serverless SQL pools
- Configure data lake security using Role-Based Access Control (RBAC) and Access Control List
Module 5: Explore, transform, and load data into the Data Warehouse using Apache Spark
This module teaches how to explore data stored in a data lake, transform the data, and load data into a relational data store. The student will explore Parquet and JSON files and use techniques to query and transform JSON files with hierarchical structures. Then the student will use Apache Spark to load data into the data warehouse and join Parquet data in the data lake with data in the dedicated SQL pool.
-
- Understand big data engineering with Apache Spark in Azure Synapse Analytics
- Ingest data with Apache Spark notebooks in Azure Synapse Analytics
- Transform data with DataFrames in Apache Spark Pools in Azure Synapse Analytics
- Integrate SQL and Apache Spark pools in Azure Synapse Analytics
Lab: Explore, transform, and load data into the Data Warehouse using Apache Spark
-
- Perform Data Exploration in Synapse Studio
- Ingest data with Spark notebooks in Azure Synapse Analytics
- Transform data with DataFrames in Spark pools in Azure Synapse Analytics
- Integrate SQL and Spark pools in Azure Synapse Analytics
Module 6: Data exploration and transformation in Azure Databricks
This module teaches how to use various Apache Spark DataFrame methods to explore and transform data in Azure Databricks. The student will learn how to perform standard DataFrame methods to explore and transform data. They will also learn how to perform more advanced tasks, such as removing duplicate data, manipulate date/time values, rename columns, and aggregate data.
-
- Describe Azure Databricks
- Read and write data in Azure Databricks
- Work with DataFrames in Azure Databricks
- Work with DataFrames advanced methods in Azure Databricks
Lab: Data Exploration and Transformation in Azure Databricks
-
- Use DataFrames in Azure Databricks to explore and filter data
- Cache a DataFrame for faster subsequent queries
- Remove duplicate data
- Manipulate date/time values
- Remove and rename DataFrame columns
- Aggregate data stored in a DataFrame
Module 7: Ingest and load data into the data warehouse
This module teaches students how to ingest data into the data warehouse through T-SQL scripts and Synapse Analytics integration pipelines. The student will learn how to load data into Synapse dedicated SQL pools with PolyBase and COPY using T-SQL. The student will also learn how to use workload management along with a Copy activity in a Azure Synapse pipeline for petabyte-scale data ingestion.
-
- Use data loading best practices in Azure Synapse Analytics
- Petabyte-scale ingestion with Azure Data Factory
Lab: Ingest and load Data into the Data Warehouse
-
- Perform petabyte-scale ingestion with Azure Synapse Pipelines
- Import data with PolyBase and COPY using T-SQL
- Use data loading best practices in Azure Synapse Analytics
Module 8: Transform data with Azure Data Factory or Azure Synapse Pipelines
This module teaches students how to build data integration pipelines to ingest from multiple data sources, transform data using mapping data flows, and perform data movement into one or more data sinks.
-
- Data integration with Azure Data Factory or Azure Synapse Pipelines
- Code-free transformation at scale with Azure Data Factory or Azure Synapse Pipelines
Lab: Transform Data with Azure Data Factory or Azure Synapse Pipelines
-
- Execute code-free transformations at scale with Azure Synapse Pipelines
- Create data pipeline to import poorly formatted CSV files
- Create Mapping Data Flows
Module 9: Orchestrate data movement and transformation in Azure Synapse Pipelines
In this module, you will learn how to create linked services, and orchestrate data movement and transformation using notebooks in Azure Synapse Pipelines.
-
- Orchestrate data movement and transformation in Azure Data Factory
Lab: Orchestrate data movement and transformation in Azure Synapse Pipelines
-
- Integrate Data from Notebooks with Azure Data Factory or Azure Synapse Pipelines
- Integrate Data from Notebooks with Azure Data Factory or Azure Synapse Pipelines
Module 10: Optimize query performance with dedicated SQL pools in Azure Synapse
In this module, students will learn strategies to optimize data storage and processing when using dedicated SQL pools in Azure Synapse Analytics. The student will know how to use developer features, such as windowing and HyperLogLog functions, use data loading best practices, and optimize and improve query performance.
-
- Optimize data warehouse query performance in Azure Synapse Analytics
- Understand data warehouse developer features of Azure Synapse Analytics
Lab: Optimize Query Performance with Dedicated SQL Pools in Azure Synapse
-
- Understand developer features of Azure Synapse Analytics
- Optimize data warehouse query performance in Azure Synapse Analytics
- Improve query performance
Module 11: Analyze and Optimize Data Warehouse Storage
In this module, students will learn how to analyze then optimize the data storage of the Azure Synapse dedicated SQL pools. The student will know techniques to understand table space usage and column store storage details. Next the student will know how to compare storage requirements between identical tables that use different data types. Finally, the student will observe the impact materialized views have when executed in place of complex queries and learn how to avoid extensive logging by optimizing delete operations.
-
- Analyze and optimize data warehouse storage in Azure Synapse Analytics
Lab: Analyze and Optimize Data Warehouse Storage
-
- Check for skewed data and space usage
- Understand column store storage details
- Study the impact of materialized views
- Explore rules for minimally logged operations
Module 12: Support Hybrid Transactional Analytical Processing (HTAP) with Azure Synapse Link
In this module, students will learn how Azure Synapse Link enables seamless connectivity of an Azure Cosmos DB account to a Synapse workspace. The student will understand how to enable and configure Synapse link, then how to query the Azure Cosmos DB analytical store using Apache Spark and SQL serverless.
-
- Design hybrid transactional and analytical processing using Azure Synapse Analytics
- Configure Azure Synapse Link with Azure Cosmos DB
- Query Azure Cosmos DB with Apache Spark pools
- Query Azure Cosmos DB with serverless SQL pools
Lab: Support Hybrid Transactional Analytical Processing (HTAP) with Azure Synapse Link
-
- Configure Azure Synapse Link with Azure Cosmos DB
- Query Azure Cosmos DB with Apache Spark for Synapse Analytics
- Query Azure Cosmos DB with serverless SQL pool for Azure Synapse Analytics
Module 13: End-to-end security with Azure Synapse Analytics
In this module, students will learn how to secure a Synapse Analytics workspace and its supporting infrastructure. The student will observe the SQL Active Directory Admin, manage IP firewall rules, manage secrets with Azure Key Vault and access those secrets through a Key Vault linked service and pipeline activities. The student will understand how to implement column-level security, row-level security, and dynamic data masking when using dedicated SQL pools.
-
- Secure a data warehouse in Azure Synapse Analytics
- Configure and manage secrets in Azure Key Vault
- Implement compliance controls for sensitive data
Lab: End-to-end security with Azure Synapse Analytics
-
- Secure Azure Synapse Analytics supporting infrastructure
- Secure the Azure Synapse Analytics workspace and managed services
- Secure Azure Synapse Analytics workspace data
Module 14: Real-time Stream Processing with Stream Analytics
In this module, students will learn how to process streaming data with Azure Stream Analytics. The student will ingest vehicle telemetry data into Event Hubs, then process that data in real time, using various windowing functions in Azure Stream Analytics. They will output the data to Azure Synapse Analytics. Finally, the student will learn how to scale the Stream Analytics job to increase throughput.
-
- Enable reliable messaging for Big Data applications using Azure Event Hubs
- Work with data streams by using Azure Stream Analytics
- Ingest data streams with Azure Stream Analytics
Lab: Real-time Stream Processing with Stream Analytics
-
- Use Stream Analytics to process real-time data from Event Hubs
- Use Stream Analytics windowing functions to build aggregates and output to Synapse Analytics
- Scale the Azure Stream Analytics job to increase throughput through partitioning
- Repartition the stream input to optimize parallelization
Module 15: Create a Stream Processing Solution with Event Hubs and Azure Databricks
In this module, students will learn how to ingest and process streaming data at scale with Event Hubs and Spark Structured Streaming in Azure Databricks. The student will learn the key features and uses of Structured Streaming. The student will implement sliding windows to aggregate over chunks of data and apply watermarking to remove stale data. Finally, the student will connect to Event Hubs to read and write streams.
-
- Process streaming data with Azure Databricks structured streaming
Lab: Create a Stream Processing Solution with Event Hubs and Azure Databricks
-
- Explore key features and uses of Structured Streaming
- Stream data from a file and write it out to a distributed file system
- Use sliding windows to aggregate over chunks of data rather than all data
- Apply watermarking to remove stale data
- Connect to Event Hubs read and write streams
Module 16: Build reports using Power BI integration with Azure Synpase Analytics
In this module, the student will learn how to integrate Power BI with their Synapse workspace to build reports in Power BI. The student will create a new data source and Power BI report in Synapse Studio. Then the student will learn how to improve query performance with materialized views and result-set caching. Finally, the student will explore the data lake with serverless SQL pools and create visualizations against that data in Power BI.
-
- Create reports with Power BI using its integration with Azure Synapse Analytics
Lab: Build reports using Power BI integration with Azure Synpase Analytics
-
- Integrate an Azure Synapse workspace and Power BI
- Optimize integration with Power BI
- Improve query performance with materialized views and result-set caching
- Visualize data with SQL serverless and create a Power BI report
Module 17: Perform Integrated Machine Learning Processes in Azure Synapse Analytics
This module explores the integrated, end-to-end Azure Machine Learning and Azure Cognitive Services experience in Azure Synapse Analytics. You will learn how to connect an Azure Synapse Analytics workspace to an Azure Machine Learning workspace using a Linked Service and then trigger an Automated ML experiment that uses data from a Spark table. You will also learn how to use trained models from Azure Machine Learning or Azure Cognitive Services to enrich data in a SQL pool table and then serve prediction results using Power BI.
-
- Use the integrated machine learning process in Azure Synapse Analytics
Lab: Perform Integrated Machine Learning Processes in Azure Synapse Analytics
-
- Create an Azure Machine Learning linked service
- Trigger an Auto ML experiment using data from a Spark table
- Enrich data using trained models
- Serve prediction results using Power BI
Successful students start this course with knowledge of cloud computing concepts and professional experience with data solutions.
Candidates must have solid knowledge of data processing languages, such as SQL, Python, or Scala, and they need to understand parallel processing and data architecture patterns.
Specifically:
- Creating cloud resources in Microsoft Azure
- Identifying use cases for big data
- Understanding how cloud compute services can solve for common business needs
To gain these prerequisite skills, take the following free online training before attending the course:
- Operating System – macOS, Windows 7 or above
- Putty software to setup SSH connection
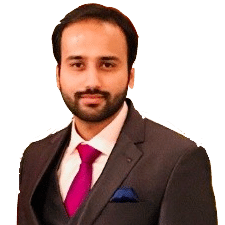
Shivam Sharma – Co-founder, TechScalable
Shivam is an author, cloud architect, speaker, and Co-Founder at TechScalable. Being passionate about ever-evolving technology he works on Azure, GCP, Machine Learning & Blockchain. He is also a Microsoft Certified Trainer.
Shivam architects’ solutions on Cloud as well on-premises using a wide array of platforms/technologies. Having core training and consulting experience, he is involved in delivering Azure and Machine Learning training to corporates like BCG, Microsoft, Intuit, RedHat, VMWare, HCL, GE, Applied Materials, Dell, Infosys, IBM, Schneider, L&T, TCS, Capgemini, Mercedes-Benz, Oracle, HP, Wipro, Colt, Cipla, LinkedIn, Mindtree.
He is certified in below:
· Microsoft Certified: Azure Administrator Associate (AZ-104)
· Microsoft Certified: Azure Solutions Architect Expert
· Microsoft Certified Trainer (MCT)
· Microsoft Certified: Azure Data Scientist Associate
· Microsoft Certified: Azure Developer Associate
· Microsoft Certified: Azure DevOps Engineer Expert
· Microsoft Certified: Azure Security Engineer Associate
· Microsoft Certified: Azure AI Engineer Associate
· Microsoft Certified: Azure Fundamentals
· MCSA: Machine Learning – Certified 2018
· MCSE: Cloud Platform and Infrastructure — Certified 2018
· MCSE: Cloud Platform and Infrastructure — Certified 2016
· Google Cloud Certified – Associate Cloud Engineer
It will be purely Hands-on and Case study driven training program.
In case the batch is cancelled, the amount would be credited back to the payee’s account in 5 working days.